By James Hyman – Syracuse University ’21
Abstract
As advanced analytics and efficiency metrics become increasingly more popular and utilized in the NBA, the WNBA is falling behind due to a lack of data and data-driven decision making. This research will specifically focus on play types, and the decisions that players make in certain isolation and post-up situations, while also incorporating a psychological aspect of offensive choices.
Introduction
The data that was used for this research was player-seasons from 2015-2020 scraped via Synergy. The first step was to investigate what aspects of play design I wanted to explore further. As seen by this graphic, isolation and post up percentage are the two largest discrepancies between the NBA and WNBA, which is what prompted those play types to be the main area of focus.
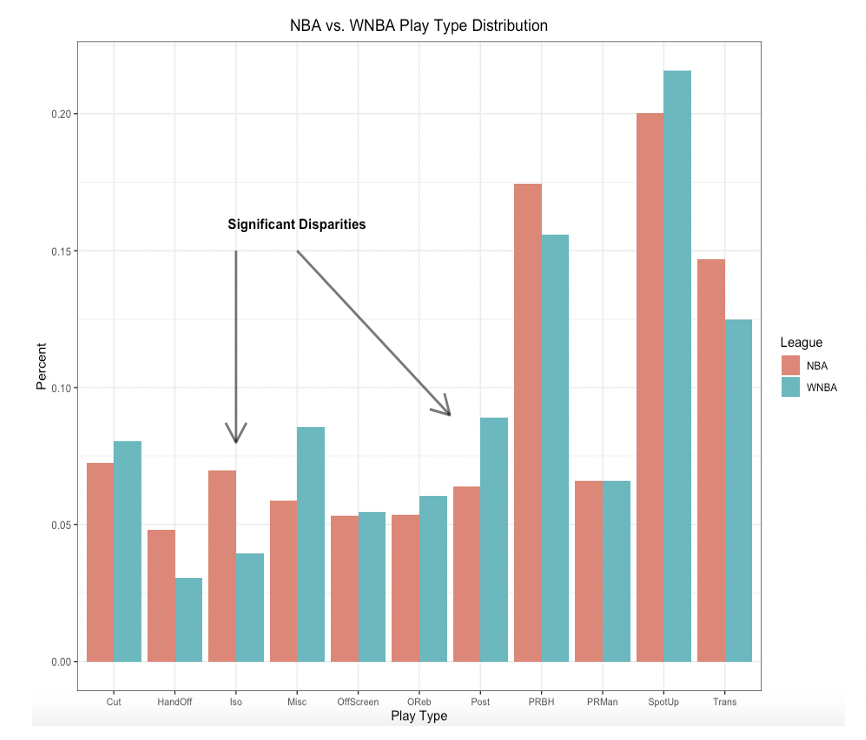
Clustering
Not all players have the same expectations when it comes to skills, therefore we cannot accurately assess decision making by evaluating everyone equally. Rather than split up by predefined positional groups which can be misleading, I used hierarchical clustering with prototypes through minimax linkage to make the groups of players. The independent variables in the clustering were the tendency (in % of time) of each player to do one of the 11 possible play types. The optimal value of k was 4, and each of the groups has a prototype player that is representative of that group’s skill type.
Cluster 1: A’ja Wilson 2019 – Traditional post-player, rarely on the perimeter
Cluster 2: Kelsey Plum 2018 – Ball dominant guard, high pick and roll percentage
Cluster 3: Riquana Williams 2020 – Off-ball guard/wing, transition and spot up specialists
Cluster 4: Candace Parker 2018 – Elite scoring forward, diverse tendencies
Methodology and Results
There were three main questions that were looked at from a regression perspective. The first was the breakdown of how success varied by these cluster groups for each play type. The results of a linear model are shown below, with some variables omitted if the play type does not explicitly apply to that skill type.
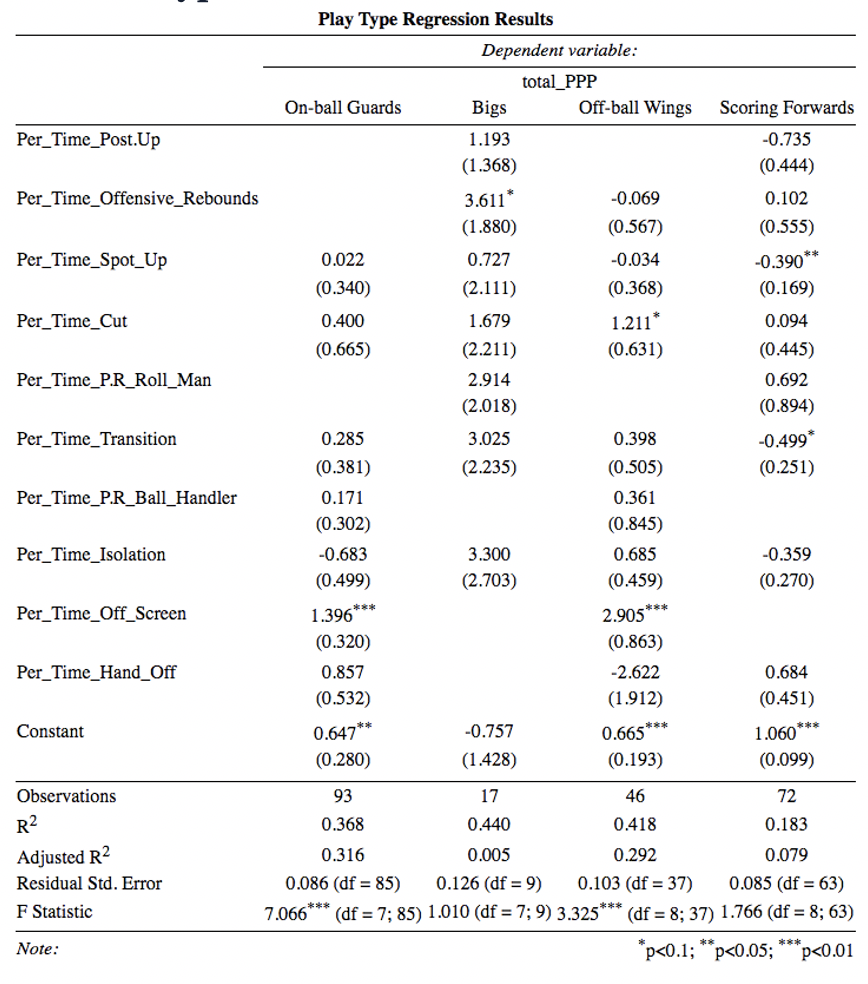
The major takeaways from this model are more coaching specific than player decision related, as an off-ball screen (significant for guards and wings) is not a choice that a player implicitly makes. Another important aspect of screens and cuts is that they are more likely to lead to baskets because the player will not be passed the ball unless they have a clear lane. The most interesting aspect of this model is the -.39 coefficient for spot up in the forward cluster. These players are so efficient at making their own shot, that it is effectively worse for their points per possession to be relying heavily on spotting up and depending on a teammate to get them an open shot.
Getting into the breakdown of individual plays, we know that isolation attempts are becoming increasingly rare, and we can see why in the efficiency graphic below.
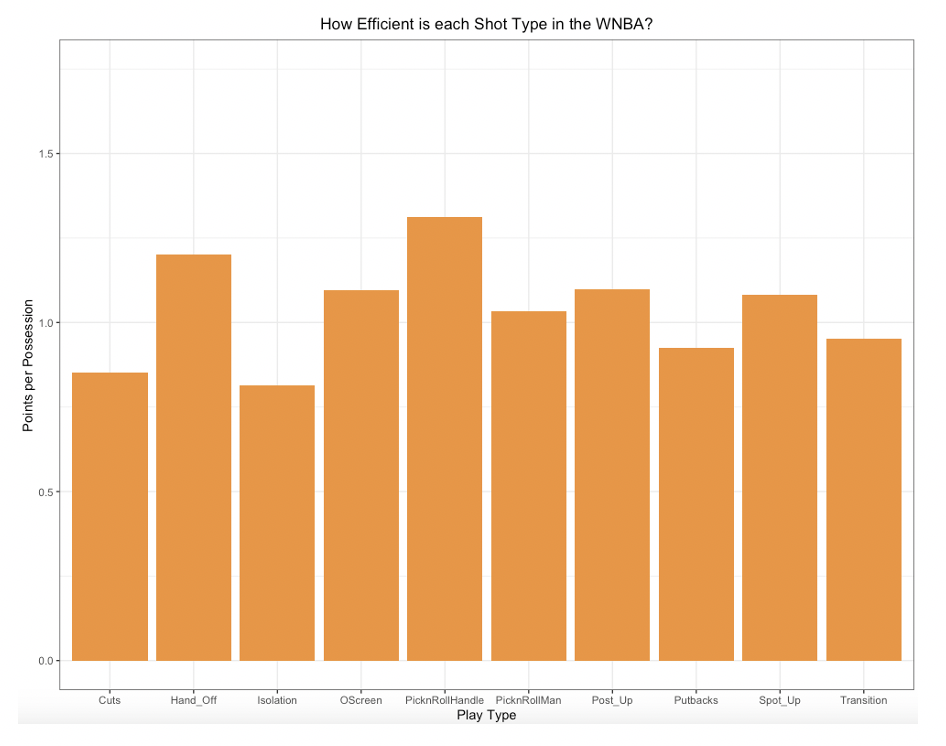
Not all isolation attempts are created equal, however, and Synergy breaks each shot into whether they drive right or left, and then whether it was a pull-up, floater, or drive to the rim. Looking at another regression model (excluding bigs who very rarely have iso attempts) we can see which decisions might be more effective than others.
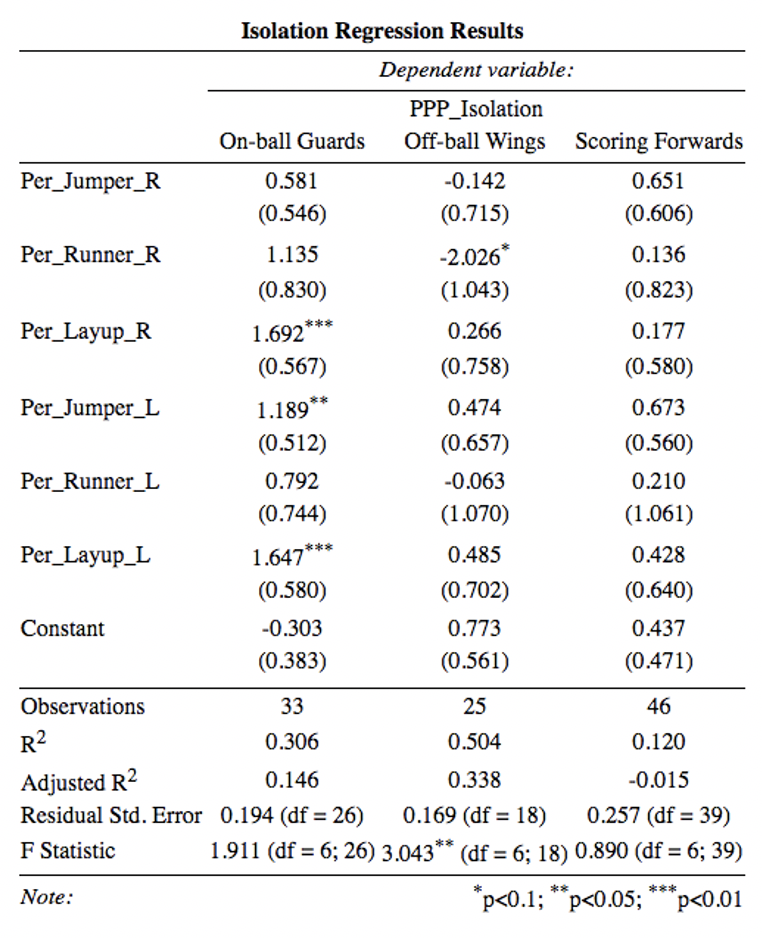
There are a few important aspects of the isolation model that are limitations of this research due to the data that exists and sample size issues. No decision to go left or right is purely based on the offensive player’s mindset, rather it can be reactionary to the defense in front of them. Since the WNBA does not utilize tracking data, it is impossible to analyze from a pure numbersperspective without film how often these choices are truly made by the offensive player. In addition, players have innate imbalances in their skill set due to factors such as their handedness. Just nine qualified players in the entire league are lefties, meaning the sample size is too small for any robust modelling. However, left-handed players do seem to have a tactical advantage as seen below by their higher average points per possession in isolation. Interestingly, both right and left-handed players do better when driving on their weak hand, an indication that players who have better control of both hands have a chance to be more efficient.
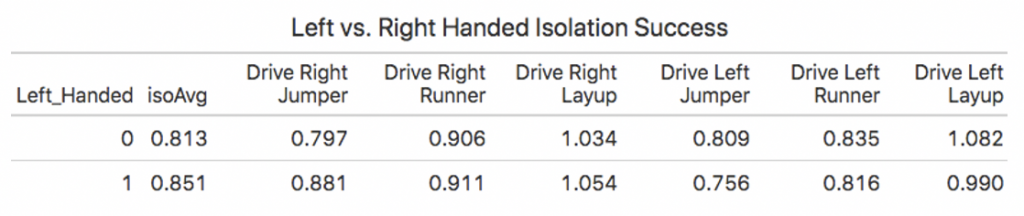
The last type of play I wanted to investigate was post-ups, since Synergy has a plethora of data on how efficient players are depending on their starting block position and the type of post movethey utilize. However, the breakdown is so in depth that it is impossible to model any individual post move decision with a reasonable sample size. Instead of looking at points per possession directly, I focused on which type of post move was most likely to lead to a player getting fouled. Foul shots are extremely efficient from an expected value perspective, as players like James Harden have shown in the NBA. Women’s players, despite being on average better free throw shooter’s than their male counterparts, are not as aggressive in their pursuit of free throw opportunities.
A specific example of this inefficiency is perennial MVP candidate Elena Delle Donne. The Mystics forward made a whopping 97% of her charity stripe attempts last season, but only shot 3.8 a game, whereas Harden gets 10+ attempts every night.
Looking into the data for field goal rates by post decision, a clear outlier was drop steps on a player’s dominant hand. Delle Donne was able to generate free throw trips almost 2X the rate of other post moves on drop steps. This applied to other notable bigs such as Brittney Griner and Tina Charles at a lesser rate. While the sample size is still small, this points again to the point of aggression as an inefficiency in women’s sports. Drop steps and other successful moves like direct drives to the basket signal a larger willingness to absorb contact for the sake of points than something like a hook shot, thus players might want to increase their attempts for post moves with efficient free-throw inducing outcomes.
Conclusions
There is very little work done on WNBA analytics, and even some of the more trivial aspects of this research are innovate in nature to the field. While purely play type tendency data alone does not tell the full story of what is going on in a player’s head, we can conclude that there are play designs and isolation/post-up actions that can increase a player’s efficiency. The next step of this research is focusing on the defensive aspect of how players react to the offensive player’s decision, with a game theory like thought process to modelling expected outcomes.
Acknowledgements
I want to thank Dr. Rodney Paul for his constant support throughout not only the thesis process but my entire academic career in Sport Analytics. None of this would be possible without him, the rest of the SAL faculty, or my peers within the program.